Machine Learning for Accountants: A Revolution is Brewing in Financial Reporting
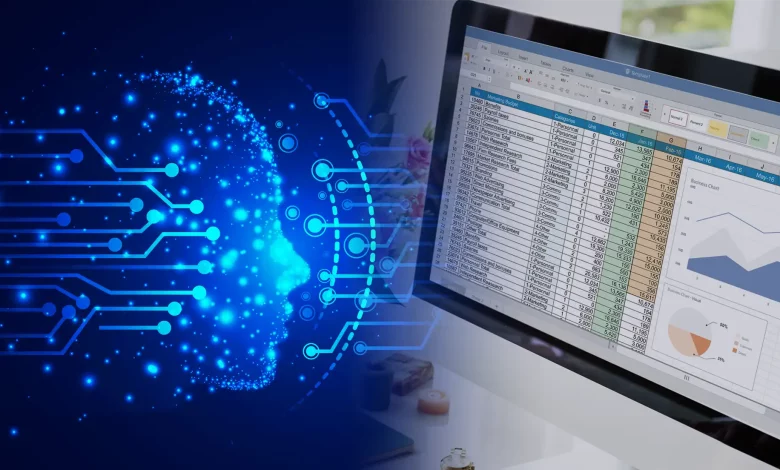
In the rapidly evolving landscape of finance and accounting, Machine Learning (ML) has emerged as a game-changing technology. This powerful subset of Artificial Intelligence (AI) is transforming how financial professionals analyze data, make decisions, and report financial information. As businesses generate unprecedented volumes of data, ML offers the tools to extract meaningful insights, automate routine tasks, and enhance decision-making processes.
This article explores how ML is reshaping the roles of accountants and finance professionals, particularly in the realms of financial reporting and decision making. We’ll delve into the fundamentals of ML, its practical applications, benefits, challenges, and future trends in the finance sector.
Basics of Machine Learning
Definition and Key Concepts
Machine Learning is a branch of AI that focuses on building systems that learn from and adapt to data without being explicitly programmed. Unlike traditional software that follows pre-defined rules, ML algorithms improve their performance as they are exposed to more data over time.
Key concepts in ML include:
- Training data: The initial dataset used to teach the algorithm
- Features: The input variables used by the model
- Labels: The output or prediction the model is trying to produce
- Model: The mathematical representation of the real-world process
Types of ML Algorithms
There are three main types of ML algorithms:
- Supervised Learning: The algorithm learns from labeled data to predict outcomes for new, unseen data. Examples include linear regression and decision trees.
- Unsupervised Learning: The algorithm finds patterns in unlabeled data. Clustering algorithms are a common example.
- Reinforcement Learning: The algorithm learns by interacting with an environment and receiving feedback on its actions.
How ML Differs from Traditional Software
Unlike traditional software, which relies on explicit programming instructions, ML systems can adapt and improve their performance over time. This flexibility allows ML to handle complex, data-intensive tasks that would be impractical or impossible to code using conventional methods.
Applications in Financial Reporting
Automated Data Entry and Processing
ML is revolutionizing data entry and processing in financial reporting. Optical Character Recognition (OCR) powered by ML can automatically extract data from invoices, automatically categorize expenses from scanned receipts, and similar data from other financial documents with high accuracy. A study by the American Institute of CPAs (AICPA) found that ML-based OCR systems can reduce data entry time by up to 80% while improving accuracy. Another case study by Intuit showed that their AI-powered bookkeeping assistant, QuickBooks Assistant, reduced the time spent on transaction categorization by 80% for small business owners.
ML algorithms are also being used to automate bank reconciliations. For example, Xero’s bank reconciliation feature uses ML to match transactions automatically, reducing the time accountants spend on this task by up to 90%.
Anomaly Detection in Financial Statements
ML algorithms excel at identifying unusual patterns or outliers in financial data. By analyzing historical financial statements, these algorithms can flag potential errors, inconsistencies, or even fraudulent activities. For example, research published in the Journal of Accounting Research demonstrated that ML models could detect financial statement fraud with over 90% accuracy, significantly outperforming traditional statistical methods.
Predictive Analytics for Financial Forecasting
ML-powered predictive analytics is enhancing the accuracy of financial forecasts. By analyzing vast amounts of historical and real-time data, ML models can predict future financial trends, cash flows, and potential risks. A report by Deloitte found that companies using ML for financial forecasting improved their prediction accuracy by 30% to 50% compared to traditional methods.
In accounting, ML is enhancing the accuracy of accrual calculations and revenue forecasting. For example, IBM’s Planning Analytics uses ML to analyze historical accounting data and generate more accurate accrual estimates, reducing the time accountants spend on this complex task.
Another application is in accounts receivable management. ML models can predict which invoices are likely to be paid late, allowing accountants to prioritize collection efforts. A study by Versapay found that companies using ML for accounts receivable predictions improved their days sales outstanding (DSO) by an average of 10%.
Streamlining Compliance and Risk Management
ML is also simplifying compliance and risk management processes. Natural Language Processing (NLP) algorithms can analyze regulatory documents and automatically update compliance requirements. Additionally, ML models can assess various risk factors in real-time, allowing for more proactive risk management. According to a survey by Thomson Reuters, 75% of financial institutions reported that AI and ML helped them enhance their compliance and risk management capabilities.
ML is also proving invaluable in tax accounting. For instance, KPMG’s ML-powered tool, KPMG Tax Reimagined, automates the extraction of relevant data from tax documents and populates tax returns, reducing processing time by up to 80%.
In audit, ML algorithms can analyze 100% of transactions rather than relying on sampling methods. Deloitte’s Argus system uses ML to read and extract information from complex documents like contracts or leases, significantly speeding up the audit process.
Enhancing Decision Making
Real-time Financial Analysis
ML enables real-time analysis of financial data, providing instant insights to support decision-making. For example, ML algorithms can continuously monitor key performance indicators (KPIs) and alert finance professionals to significant changes or trends. For example, an ML system could continuously monitor the current ratio, debt-to-equity ratio, or inventory turnover, alerting accountants to significant changes that might require attention.
This real-time capability allows for more agile and responsive financial management.
Improved Fraud Detection
ML algorithms are particularly adept at detecting fraudulent activities. By analyzing patterns in transactional data, these systems can identify suspicious behaviors that might escape human detection. A study published in the Journal of Banking and Finance found that ML-based fraud detection systems could identify up to 95% of fraudulent transactions, significantly outperforming rule-based systems.
In accounts payable, ML algorithms can detect potential duplicate payments or unusual spending patterns.
Personalized Financial Advice and Recommendations
In wealth management and personal finance, ML is enabling more personalized financial advice. By analyzing an individual’s financial history, risk tolerance, and market conditions, ML algorithms can generate tailored investment recommendations.
For management accountants, ML can provide tailored cost-saving recommendations. For example, an ML system might analyze production costs and suggest optimal inventory levels to minimize holding costs while avoiding stockouts.
Optimizing Investment Strategies
For institutional investors and fund managers, ML is revolutionizing investment strategies. ML algorithms can analyze vast amounts of market data, news sentiment, and economic indicators to identify investment opportunities and optimize portfolio allocation. A study by the CFA Institute found that 71% of investment professionals expect AI and ML to have a significant impact on their investment decision-making process within the next 10 years.
While more common in finance, this also applies to accountants involved in treasury management. ML models can optimize cash management strategies, balancing factors like liquidity needs, interest rates, and foreign exchange risks.
Benefits for Accountants and Finance Professionals
Increased Efficiency and Productivity
One of the most significant benefits of ML for finance professionals is the dramatic increase in efficiency and productivity. By automating routine tasks such as data entry, reconciliation, and report generation, ML frees up valuable time for higher-level analysis and strategic planning. A report by Accenture estimated that AI and ML could increase labor productivity in the finance sector by up to 40% by 2035.
ML is dramatically reducing the time accountants spend on routine tasks. For example, BlackLine’s ML-powered transaction matching can automate up to 80% of high-volume reconciliations, freeing accountants to focus on investigating discrepancies and higher-value analysis.
Enhanced Accuracy and Reduced Errors
ML algorithms can process vast amounts of data with a level of accuracy that surpasses human capabilities. This not only reduces errors in financial reporting but also enhances the overall quality of financial analysis. A study published in the Journal of Accounting and Economics found that financial statements prepared with the assistance of ML had 37% fewer errors compared to those prepared manually.
ML can significantly reduce errors in complex accounting calculations. For instance, in lease accounting, ML algorithms can more accurately classify leases and calculate right-of-use assets and lease liabilities, ensuring compliance with standards like ASC 842 and IFRS 16.
More Time for Strategic Analysis and Planning
As ML takes over routine tasks, accountants and finance professionals can dedicate more time to strategic analysis and planning. For example, instead of spending hours reconciling accounts, accountants can use that time to perform profitability analysis by product line or customer segment, providing valuable input for business strategy. This shift allows them to provide more value-added services to their organizations or clients. According to a survey by the Institute of Management Accountants, 76% of finance professionals believe that AI and ML will allow them to focus more on strategic initiatives.
Improved Client Services
For accounting and financial advisory firms, ML enables the provision of more sophisticated and personalized client services. For instance, an ML system could analyze a client’s financial data and alert the accountant to potential issues, such as an impending cash flow problem or an unusual increase in expenses, allowing for timely advice. From real-time financial dashboards to AI-powered chatbots for basic financial queries, ML is enhancing client engagement and satisfaction.
Challenges and Considerations
Data Quality and Availability
The effectiveness of ML models heavily depends on the quality and quantity of available data. Finance professionals must ensure they have access to clean, comprehensive, and relevant data to train ML algorithms effectively.
In accounting, ensuring the quality and consistency of data across different systems (e.g., ERP, CRM, and legacy systems) is crucial for effective ML implementation. Accountants may need to work closely with IT teams to ensure data integrity and accessibility.
Ethical Considerations and Bias in ML Models
As ML models increasingly influence financial decisions, it’s crucial for accountants to be aware of potential biases in ML models. For instance, a credit scoring model used for accounts receivable management must be carefully designed to avoid unfair treatment of certain customer groups.
The European Union’s General Data Protection Regulation (GDPR) and similar regulations worldwide are addressing these concerns, requiring transparency and fairness in automated decision-making systems.
Need for Upskilling and Adaptation
Accountants will need to develop new skills to work effectively with ML systems. This might include basic data science concepts, understanding of ML outputs, and enhanced analytical skills to interpret ML-generated insights. A report by the World Economic Forum predicts that by 2025, 50% of all employees will need reskilling as adoption of technology increases.
Integration with Existing Systems
Integrating ML solutions with legacy financial systems can be challenging. Many accounting departments face challenges integrating ML solutions with existing accounting software and ERP systems. Ensuring seamless data flow between these systems is crucial for realizing the full benefits of ML.
Future Trends
Natural Language Processing for Financial Document Analysis
Advanced NLP techniques are set to revolutionize the analysis of financial documents. From automatically generating financial reports to extracting key insights from earnings calls, NLP will significantly enhance the efficiency of financial analysis. Research by OpenAI demonstrates that large language models can perform complex financial analysis tasks with near-human accuracy.
Advanced Predictive Modeling for Risk Assessment
Future ML models will offer even more sophisticated risk assessment capabilities. By incorporating a wider range of data sources and using more complex algorithms, these models will provide more accurate and nuanced risk predictions. The Bank for International Settlements predicts that such advanced ML models will play a crucial role in maintaining financial stability in the coming years.
AI-powered Virtual Financial Assistants
Virtual financial assistants powered by ML are expected to become more prevalent. These AI assistants will be capable of providing real-time financial advice, answering complex queries, and even executing financial transactions. According to a report by Business Insider Intelligence, 64% of financial services executives believe that conversational AI will be a key technology in the future of finance.
Blockchain Integration with ML for Enhanced Security
The integration of blockchain technology with ML is expected to enhance the security and transparency of financial transactions. ML algorithms can analyze blockchain data to detect fraudulent activities, while blockchain can provide a secure and immutable record of ML model outputs. A study by the IEEE predicts that this integration will be particularly impactful in areas such as supply chain finance and cross-border payments.
Conclusion
Machine Learning is undeniably transforming the landscape of financial reporting and decision making. By automating routine tasks, enhancing accuracy, and providing deeper insights, ML is empowering accountants and finance professionals to deliver more value to their organizations and clients.
However, the adoption of ML in finance also brings challenges. Ensuring data quality, addressing ethical concerns, and upskilling the workforce are crucial steps in harnessing the full potential of this technology.
As we look to the future, it’s clear that ML will continue to play an increasingly important role in accounting and finance. From advanced predictive modeling to AI-powered virtual assistants, the possibilities are vast and exciting. For accountants specifically, the integration of ML into daily practices represents both a challenge and an opportunity. While it may change the nature of many traditional accounting tasks, it also opens up new avenues for accountants to add value. By leveraging ML, accountants can move beyond number-crunching to become strategic advisors, using ML-generated insights to guide business decisions and strategy.
The future of accounting lies not just in recording financial transactions, but in interpreting and acting upon the wealth of financial data that organizations generate. Machine Learning is the key that will unlock this potential, allowing accountants to play an even more crucial role in driving business success.
As we conclude, it’s important to note that while ML offers powerful tools, it cannot replace human judgment and expertise. The most successful finance professionals of the future will be those who can effectively combine their domain knowledge with ML capabilities, using technology to augment their skills and deliver superior financial insights and decisions.
By Asif Mehdi CPA, CTP, CISA.